13 min read
An Analysis of ChatGPT and OpenAI GPT-3: How to Use it For Your Business – Version 1
Read time: 14 mins
Before you head into our analysis of Chat GPT3, as of March 14th, OpenAI have released Chat GPT-4. GPT-4 has taken the AI world by storm especially after Bing AI announced that it had started using GPT-4. Read our analysis on OpenAI’s GPT-4 where we talk about its latest features, pricing, availability, and business use cases.
You must have seen a lot of talk online recently about ChatGPT and OpenAI GPT-3. If you’re not up to speed and wondering what ChatGPT is and how to use it, then you’re in the right place. In this blog we will provide a high-level review and comparison of ChatGPT versus GPT-3 and the other AI models available in the industry to keep you informed on the latest innovations in tech, so that you can realise the business benefits of both and make the right decision for your company.
- What are GPT-3 and ChatGPT ?
- How to Use ChatGPT and GPT-3?
- ChatGPT vs GPT-3
- GPT-3 (OpenAI API) Costs
- Limitations of GPT-3
- Examples of ChatGPT
- GPT-3 vs Older Transformers Models (incl. GPT-2, BART etc.)
- ChatGPT and OpenAI ChatGPT-3 Applications
- Conclusions and Next Steps
What are GPT-3 and ChatGPT?
Both GPT-3 and ChatGPT are language models developed by OpenAI. ChatGPT is a Chatbot built on top of GPT-3 that is specifically optimised for conversational text generation, such as those in chatbots and dialogue systems. It is still in the research phase and OpenAI is rolling out the API region wise (currently available in the US).
On the other hand, GPT-3 is accessible via OpenAI’s API and is capable of Natural Language Processing (NLP) tasks such as text completion, translation, and summarisation. It is a language model, but it does not have the ability to remember past conversations, it can only learn the language from a domain-specific corpus.
Both models were trained on vast amounts of data sourced from the internet, including human conversations, allowing the model to sound human-like while generating text. The data used to train language models like GPT-3 and ChatGPT most likely includes a variety of sources, like human interactions from various platforms such as Reddit, Facebook, and others. However, the specific data sources and training methods used for these models are not always publicly disclosed.
In the case of ChatGPT, the training process was augmented by human AI trainers who provided conversations in which they played both sides – the user and the AI assistant. This was done to help the model better understand the context and tone of human conversations.
It is important to keep in mind that while these models are designed to generate human-like text, they are not perfect, and their outputs should not be taken as truth without further validation. This is because the models are based on statistical probabilities and may generate text that is not accurate or relevant to the given context.
By default, OpenAI uses data fed into its API for model improvement. It provides an option for the user to opt out of having content used for model improvement by contacting them via email. The OpenAI GPT-3 models are more expensive to run and are fine-tuned compared to older models such as GPT-2 or BART, but not necessarily unaffordable.
This would only prove useful in business scenarios where high-quality text is of value. Fine-tuning a model for question answering would involve providing a dataset that contains an input context, question, and an answer. This allows the model to learn from patterns provided by the dataset which could include domain knowledge, the format of the answers etc. Given this is a transformer model also known as the foundational model we cannot determine what exactly the model learns but it learns to predict the most probable word given a set of words.
As mentioned earlier for language models, fine-tuning a model might allow the model to sound plausible but without providing a context (a text which contains the answer for the question you are looking for) the answer provided by the model could be false.
GPT-3 models and other transformer-based models have an inherent limitation of the number of tokens they can process in one go. Tokens are pieces of words used for natural language processing (NLP). For text in English, 1 token is approximately 4 characters or 0.75 words. For comparison, Harry Potter and the Order of The Phoenix is about 250,000 words, and 185,000 tokens. The most powerful GPT-3 model DaVinci, supports a maximum of 4,000 tokens (approximately 3,000 words) including input and output which limits any business use case to this size. Both GPT-3 and other transformer methodologies have these limitations – anything large scale including text beyond the token limitation of these models would require additional research and implementation to see how they perform.
Version’s Innovation Labs co-creation team have created Smart Text which overcomes the token limitation by dividing and combining larger documents. The approach is like this research on summarising books with human feedback which was published by OpenAI.
How to Use ChatGPT and GPT-3?
Currently trivial applications of ChatGPT are based on chatbots, summarisation tools etc. Even though these cannot be commercialised until the API is available, ChatGPT could be used for:
- Generating templates for product launches for marketing
- Generating worksheets and quiz questions for schools
- Summarising emails or letters into bullet points
ChatGPT performing as a research preview has limitations in terms of commercialisation, but GPT-3 API could be commercialised for small tasks such as:
- Automated email subject creation
- Keyword generation
- Summarisation of video transcripts etc.
Some commercially viable ideas such as Automated Transcription of Audio/Video Files need further exploration in terms of how they could help businesses.
Apart from these, the API could most likely be deployed in business scenarios where data trends can be summarised through the generation of text. This would help with content creation from horizon scanning tools (like The Intelligencer, a trend tracker currently being developed internally by the Version 1 AI Labs Co-creation team) presenting personalised content from internet data.
OpenAI also has a code generating and explanatory model which could be useful for code explanation, documentation and even learning. The Version 1 Innovation Labs team will publish a report on this shortly.
ChatGPT VS GPT-3?
ChatGPT is still in its research phase and OpenAI does not provide any API. While the ability of ChatGPT to generate human-like text has garnered a lot of curiosity, and has been widely discussed across the media, applications of this model are still limited.
GPT-3 however is accessible via OpenAI API and is capable of Natural Language Processing (NLP) tasks such as text completion, translation, summarisation etc. It is a language model and does not have the capability to remember past conversations, only to learn the language from a domain-specific corpus. As mentioned previously, this means that factual responses could be made up.
Note: Both models were trained on vast amounts of data sourced from the internet, including human conversations allowing the model to sound human-like while generating text. However, this is just a result of the system’s design to maximise similarity between output and the dataset, and could in fact be producing inaccurate, inherited, biassed or even untruthful outputs.
Some examples of where GPT-3 is used now are:
- Algolia uses GPT-3 in their Algolia Answers product to offer relevant, lightning-fast semantic search for their customers. When the OpenAI API launched, Algolia partnered with OpenAI to integrate GPT-3 with their advanced search technology to create their new Answers product that better understands customers’ questions and connects them to the specific part of the content that answers their questions.
- Fable Studio is creating a new genre of interactive stories and using GPT-3 to help power their story-driven “Virtual Beings.” GPT-3 has given us the ability to give their characters life.
- Viable helps companies better understand their customers by using GPT-3 to provide useful insights from customer feedback in easy-to-understand summaries.
GPT-3 (OpenAI API) Costs?
Pricing is based on tokens and is per one thousand tokens. OpenAI estimates around 750 words form roughly one thousand tokens. A paragraph consists of thirty-five tokens.
OpenAI provides base models for implementation via it’s API.
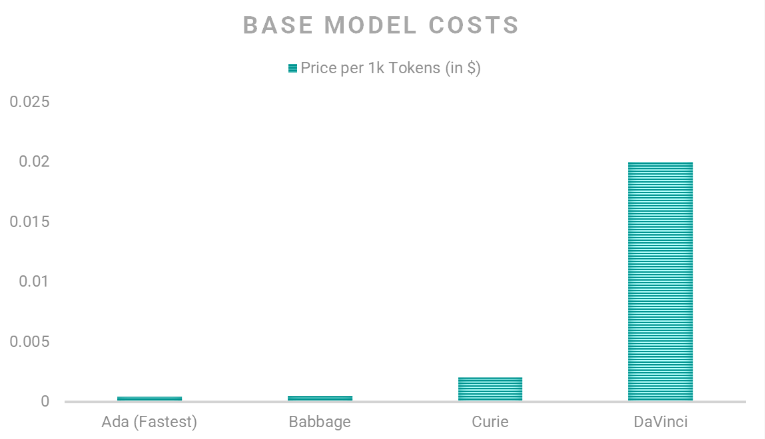
However, if you want to fine-tune a model for a specific domain, the following cost plan applies:
Limitations of GPT-3?
Whilst there are many benefits to GPT-3, it’s important to know the limitations of GPT-3 before reaching any conclusions.
Token Limitations
One of the main limitations of transformers is the number of tokens that they can process at once. When training or using a transformer-based language model, the input text is first tokenised and then converted into numerical representations (called “embeddings”) that can be processed by the model.
The number of tokens that a transformer can process at once is determined by the size of the “context window” that the model uses. A context window is a set of tokens around a target token that the model considers when making predictions about that token. The larger the context window, the more tokens the model needs to consider, and the more memory and computation is required. The size of the context window is determined by the memory constraints of the GPUs used in the experiments. GPT-3, on the other hand, can process much longer input, but the limitation may depend on the compute resources, or token-batch size as well.
Because of this token limitation, one of the common approaches to process longer text is to break it up into smaller chunks and then process each chunk separately, a process called ‘truncation.’
Business Limitations on Token Size
Most transformers support 512 tokens apart from a few which allow up to 4,096 tokens. This means any text (input) given to a transformer should be around three thousand words. Anything beyond this could be ignored or broken into multiple segments and combined later.
For example: If you want to summarise a document consisting of one hundred pages with 750 words in each page, you will need to summarise each page separately and combine them to generate a summary of summaries.
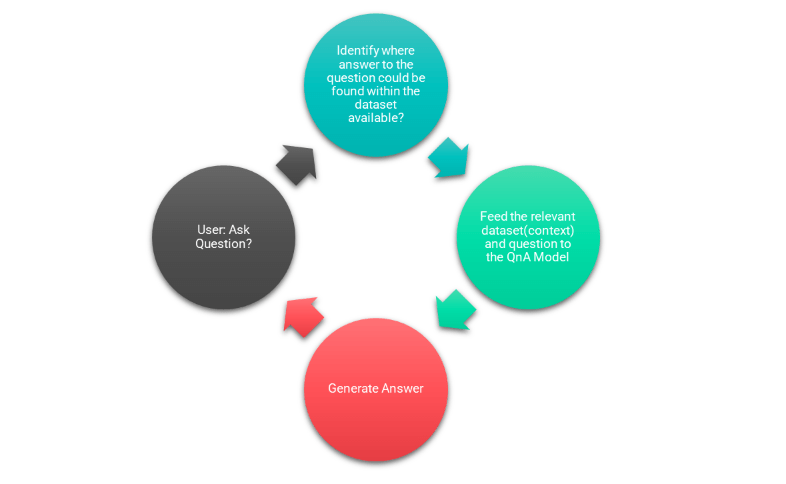
Remembering (Retaining) Information/Data
GPT-3 does not have the ability to remember specific input data, it only uses the input as a prompt for the next prediction. Therefore, after the task or session is done, GPT-3 will not retain any memory of the specific data you fed into it, but it will be able to use the general knowledge learned from previous interactions and data it was trained on to produce relevant outputs.
For example: If you want to build a question-answer system on Version 1 data, training the model just based on Version 1 data would help the model get some general knowledge and domain-based knowledge to improve the language model. However, these results could be untrue. To build a good Q&A system, the context would always have to be provided.
Possible design of Q&A system
Context based Q&A systems normally consist of the model which generates the answer and a search system which retrieves semantically related content and feeds into the model along with the question to generate the output.
Examples of GPT-3?
Let’s look at some further examples of ChatGPT at work.
Q&A
Analysis using ChatGPT
Data is sourced from publicly available data from version1.com and Wikipedia. This data is fed in as the context to both ChatGPT and a Longformer Model from Hugging Face. The Longformer was chosen since it would accept 4,096 tokens. What ChatGPT gave us:
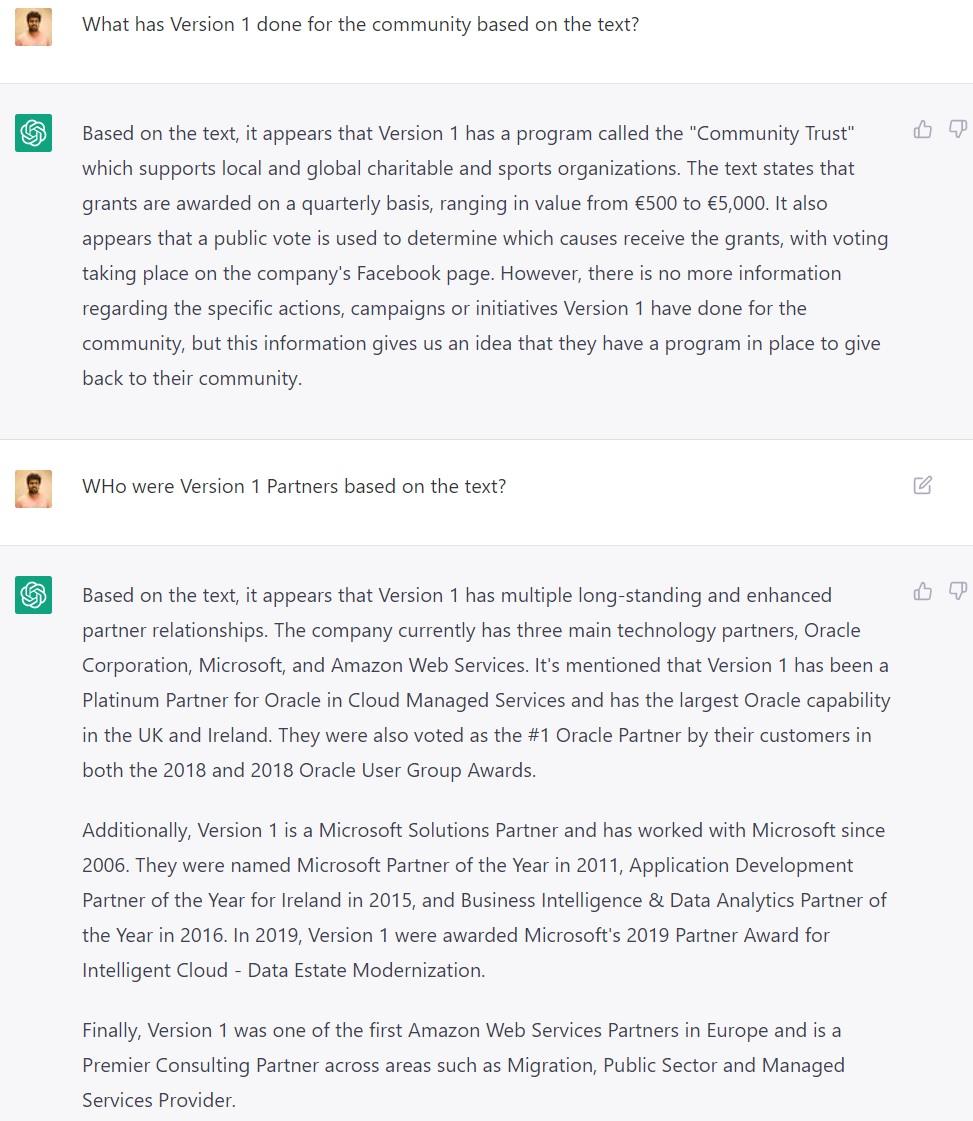
It was able to generate a sentence based on the information provided to it. However, do note the amount of data and questions that can be fed into the models and the answer it provides is restricted to 4,096 tokens.
Analysis Using Longformer Transformer Models
The below output was used with a Longformer model.
The Longformer or older transformer models on the other hand do an extractive Q&A retrieving substrings or sentences which are semantically correct to the question being asked. They do not generate perfect sentences and can only be used as a guide to fill out any answers. This means if you require “Who is Bob?” from a piece of text, the text should contain the answer “Bob is a plumber. He is 30 years old and works for Bob’s plumbing Company.” The input (context for the question) provided to the model should contain the answer to the question asked for the model to identify the correct response.
Analysis of Unemployment Rate using ChatGPT
A dataset (from kaggle.com ) containing US unemployment rates was fed to ChatGPT and asked to draft an article.
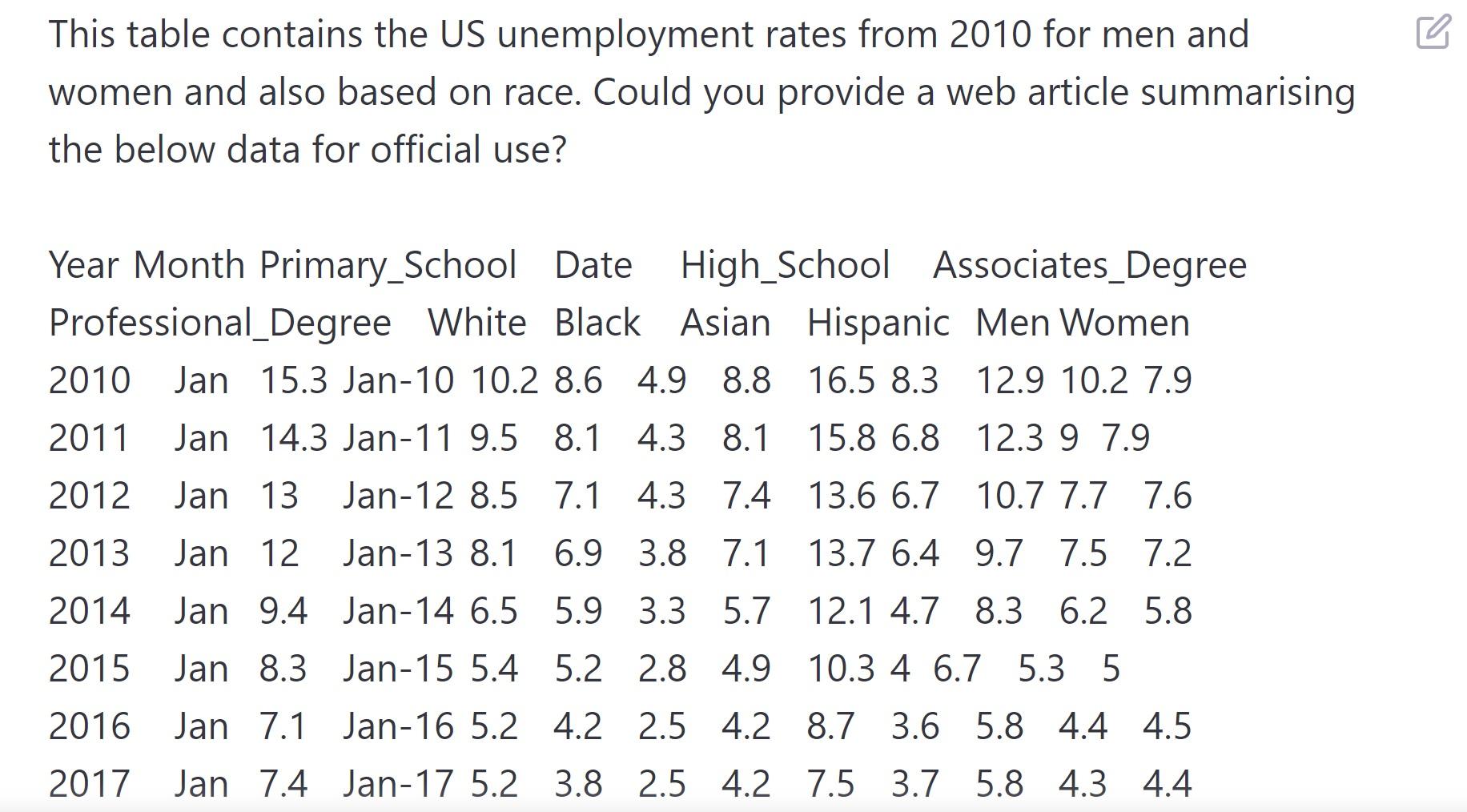
What we can get from this:
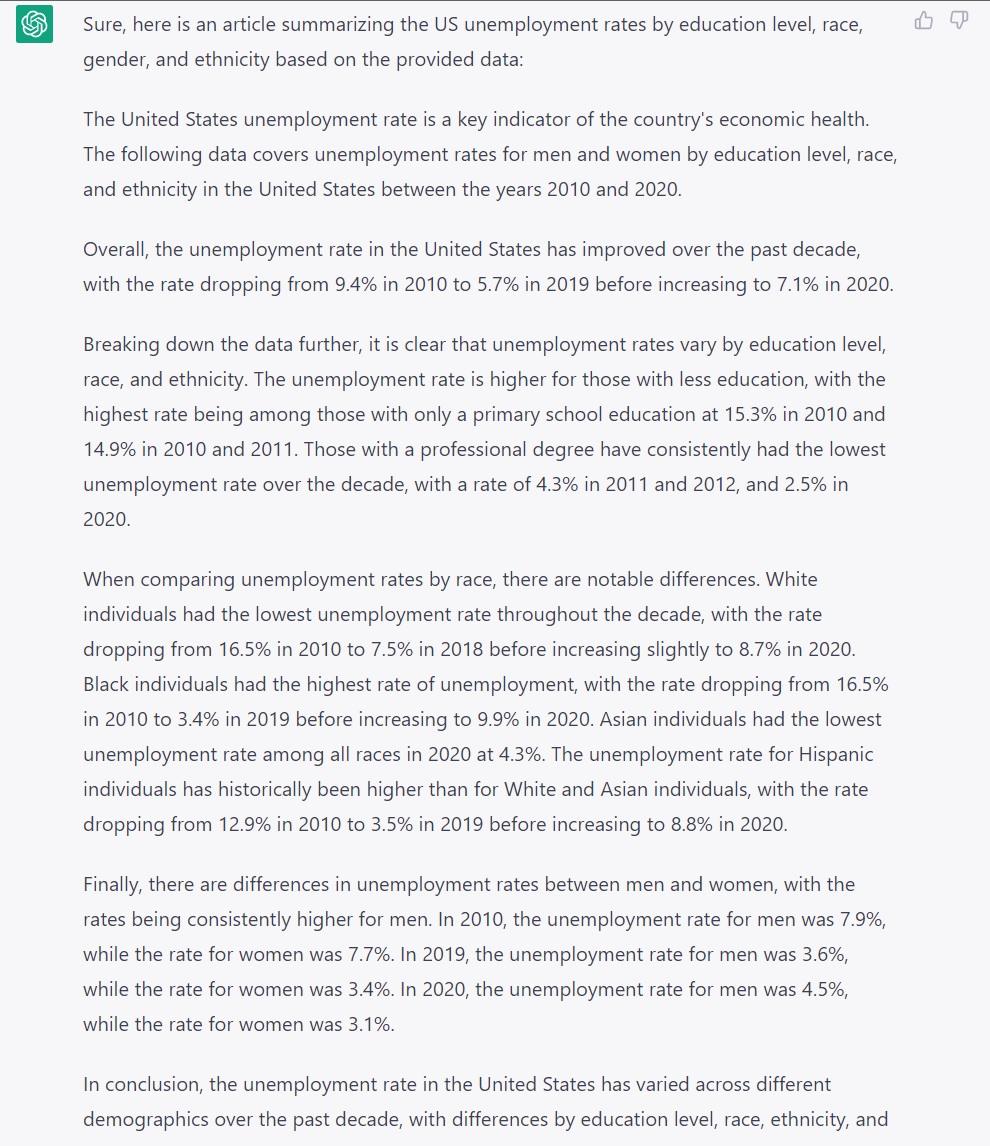
ChatGPT can identify data and statistical values and insights based on the input provided however it might not be able to analyse and provide trends unless it is provided in the input.
GPT-3 vs Older Transformer Models (incl. GPT-2, BART etc.)
The following table shows high-level differences in what OpenAI offers and what we have at present with older models such as GPT2 or BERT/BART:
ChatGPT and OpenAI ChatGPT-3 Potential Applications
Despite all the recent advancements, ChatGPT may still not be the right option for you. There are other options the Version 1 team could help you design with additional research and development.
Recommendation System Using Embedding
Given an intranet or dataset, we could generate embeddings for the dataset and use that to build a recommendation system. For example, a basic recommender model that uses nearest neighbour search.
Say you are a news company, and you want to recommend related articles for your reader. This would mean we need to generate embeddings for each article which are representations of the text within the article. This representation could be compared to recommend other similar articles, once access to the body of documents from the business is available.
Automated Transcription of Audio/Video Files
OpenAI has an Open-Source Neural Network model called Whisper which performs well on English speech recognition. The idea is to use Whisper to automatically transcribe audio files and generate a transcript for these files. These could be for the following purposes using GPT-3:
– Summarisation of Video transcripts (Used for a general description of the video)
– Video Classification through Keyword generation (Embeddings).
– Video Recommendation through Keywords (Embeddings).
Note: For video files we would need to extract the audio from the video.
Summarisation of Trends for Data Scraping/Horizon Scanning Applications
This is the most viable business use case we have identified. OpenAI API could be used in a system to generate text that summarises trends or information available in data.
For example: We have data on which jobs are trending on Indeed for the last 3 months in a tabular and graphical form. We could use OpenAI APIs to generate articles or pieces of text which could be used to develop a report or a website.
At the Version 1 AI Labs, we are currently developing a horizon scanning tool called The Intelligencer to identify technology trends in the market. We would be using data scrapped from various sources and generating visual graphs to display trends in the market. Additionally, the OpenAI API will be used to generate summarised text for these trends which will be added along with the visuals on the platform.
Conclusion and Next Steps
Older transformer models such as GPT2/BART or GPT-3 language generation models should be considered as such. Given enough training data, they can generate properly formed sentences but the outputs might not always be true unless full context is provided.
GPT-3 models might provide better language generation but are costlier to run and fine-tune compared to older foundational models and add a dependency on data being sent across the API. This would only prove useful in business scenarios where high-quality text is of value. Older transformers available on Hugging Face provide language generation but on a smaller scale and at a higher cost.
Transformer based models have limitations mentioned earlier such as token limits which could impact on scalability for business needs. Developing systems which would help users identify a particular area in the document or get shorter summaries could be implemented directly using these models. However, anything large scale including text beyond the token limitation of these models would require additional research and implementation using multiple systems to see how they perform.
In conclusion, OpenAI API has a number of viable uses for businesses, particularly for generating text that summarises trends or information from a data set. For example, by using the API to analyse job trends on Indeed, businesses can develop reports or blogs that provide insights on trending positions. This is the area that we see the most potential in and we’ll continue to report on the progress for our proof of concept, Intelligencer, on our Insights updates.
ChatGPT on the other hand seems to be able to store and retrieve data on a conversational basis and could have business use cases which are limited by the currently available technologies. However OpenAI has not revealed the underlying architecture of ChatGPT to help us understand how this system works. They have recently announced access (join waitlist here) to a commercial version of ChatGPT known as ChatGPT professional. Once released, depending on the features and limitations it provides, potential business scenarios where remembering data fed into the system could be possible.
AI also has a code generating and explanatory model which could be useful for code explanation, documentation and even learning. We are currently analysing this feature and a report on this will be published shortly. As part of the next step, we will be also looking at other emerging models of equivalent size or complexity and analysing how they perform against DaVinci.